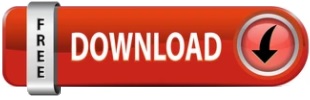
However, due to the limitations of the gradient-based optimization methods, the metaheuristic algorithms including the evolutionary algorithms, swarm intelligence, etc., are still being widely explored by the researchers aiming to obtain generalized FNN for a given problem. Its success is evident from the FNN's application to numerous real-world problems. The gradient-descent algorithm such as backpropagation has been widely applied to optimize the FNNs.
/cdn.vox-cdn.com/uploads/chorus_image/image/53166565/494203092.0.jpg)
Researchers adopted such different viewpoints mainly to improve the FNN's generalization ability. The FNN optimization is often viewed from the various perspectives: the optimization of weights, network architecture, activation nodes, learning parameters, learning environment, etc. Over the past two decades, the feedforward neural network (FNN) optimization has been a key interest among the researchers and practitioners of multiple disciplines.
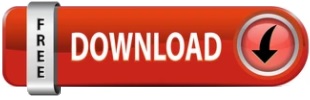